Webinar
Deep Dive: Visual Pipelines Webinar
Jul 20, 2023
Recently, we launched Visual Pipelines – a single, point-and-click interface to manage your observability pipelines.<br /> <br /> – Different use cases for Visual Pipelines, including building, testing, and monitoring pipelines<br /> – How the feature will drive developer self-service and autonomy<br /> – What’s next for the Edge Delta Observability Pipelines product
More Videos
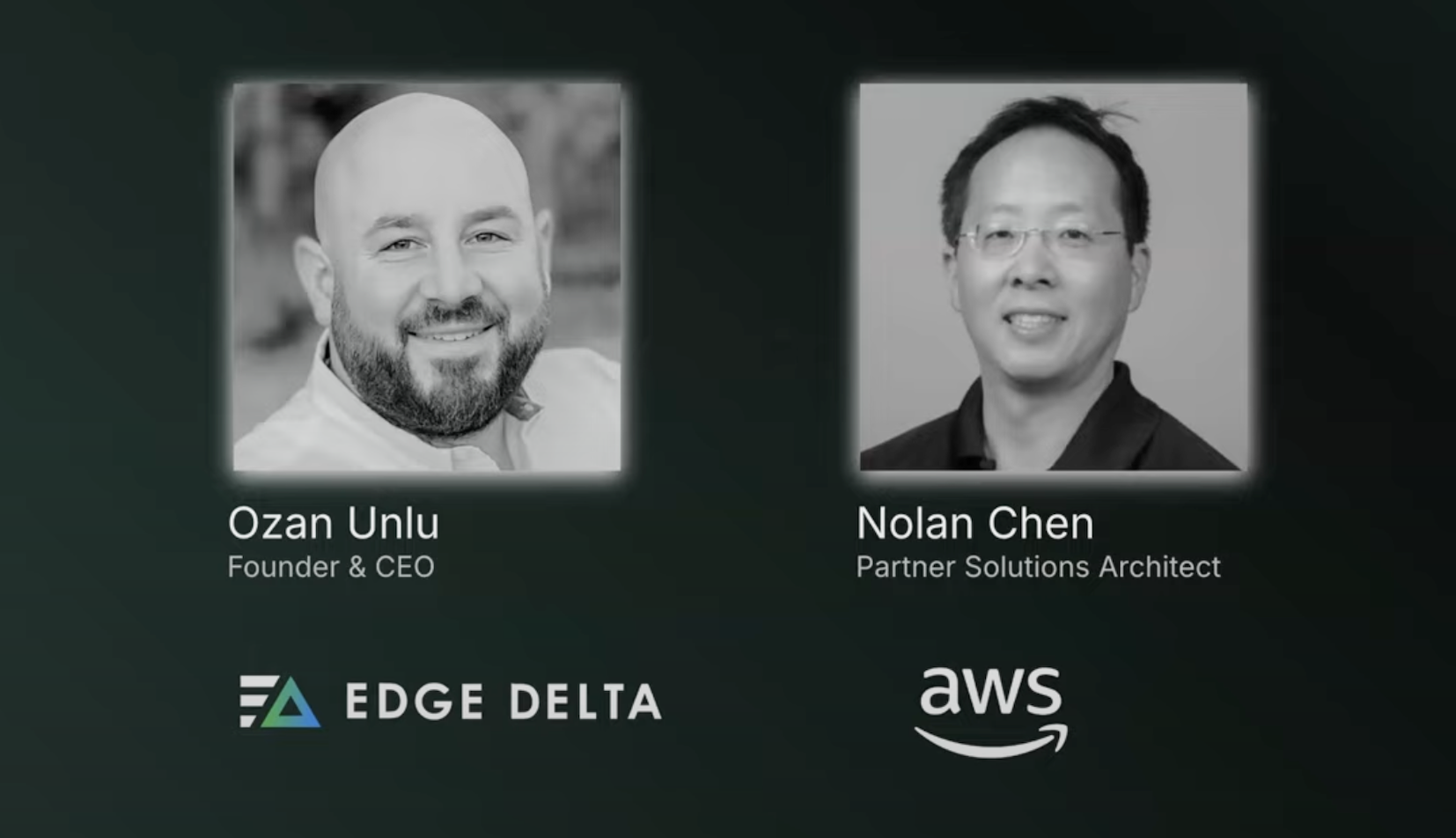
Webinar
Chaos to Control: Telemetry Pipelines for all Your Observability and Security Data
Jul 20, 2023
•
1:00:59
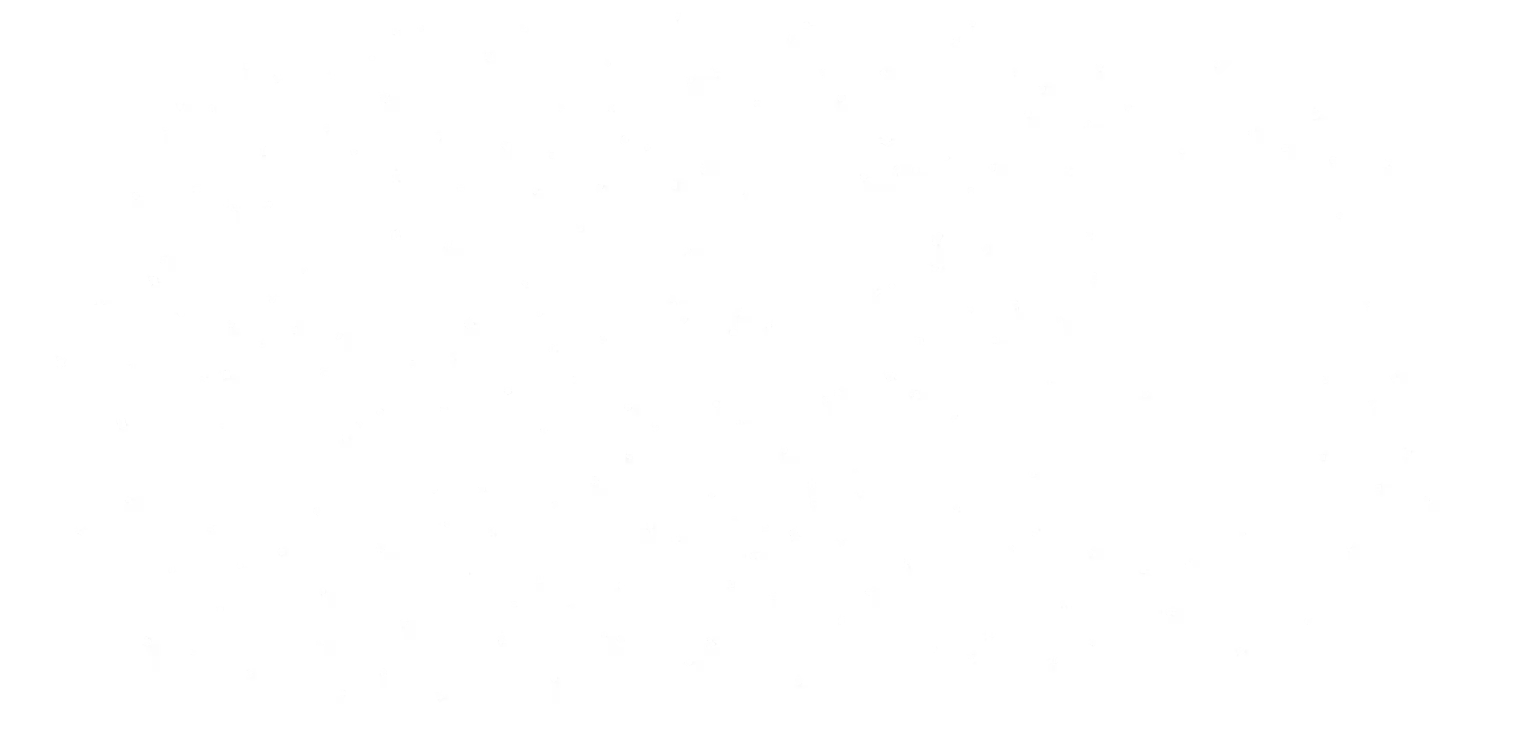