In October 2021, Splunk announced its new Workload Pricing model (sometimes referred to as Splunk Virtual Compute pricing). Splunk Workload Pricing is an alternative to volume-based pricing.
Volume-based pricing can be problematic for some customers considering the rate log data has exploded. According to a recent survey commissioned by Edge Delta, 36% of organizations generate more than 1TB of log data every day. As a byproduct of this growth, 98% reported facing overages at least a few times a year, and 82% say they limit data ingestion as a way to reduce costs.
By launching workload-based pricing, Splunk is trying to give its customers more flexibility. However, that does not mean you should expect to pay less – or even ingest more data – by switching to Workload Pricing.
Breaking Down the SVC Pricing Model
The Splunk Workload Pricing Model is based on two components:
- Splunk Virtual Compute (SVC): The compute, I/O, and memory required to monitor your data sources.
- Storage Blocks: The storage volume required to fulfill your data retention policies.
SVCs are the equivalent of vCPUs for Splunk Cloud consumption. The factors that have the biggest impact on these areas are data volume, ad hoc queries, and scheduled queries to populate dashboards and trigger alerts.
When you engage Splunk, their team will assign a predetermined amount of Splunk SVC unit credits. This amount is based on your aggregate data sources and how you use the data in Splunk:
- A dataset that isn’t queried frequently will have a higher ingestion-to-SVC ratio. For example, compliance storage or a data lake.
- A dataset that is monitored with scheduled queries and real-time dashboards will have a lower ingestion-to-SVC ratio. For example, security or service monitoring.
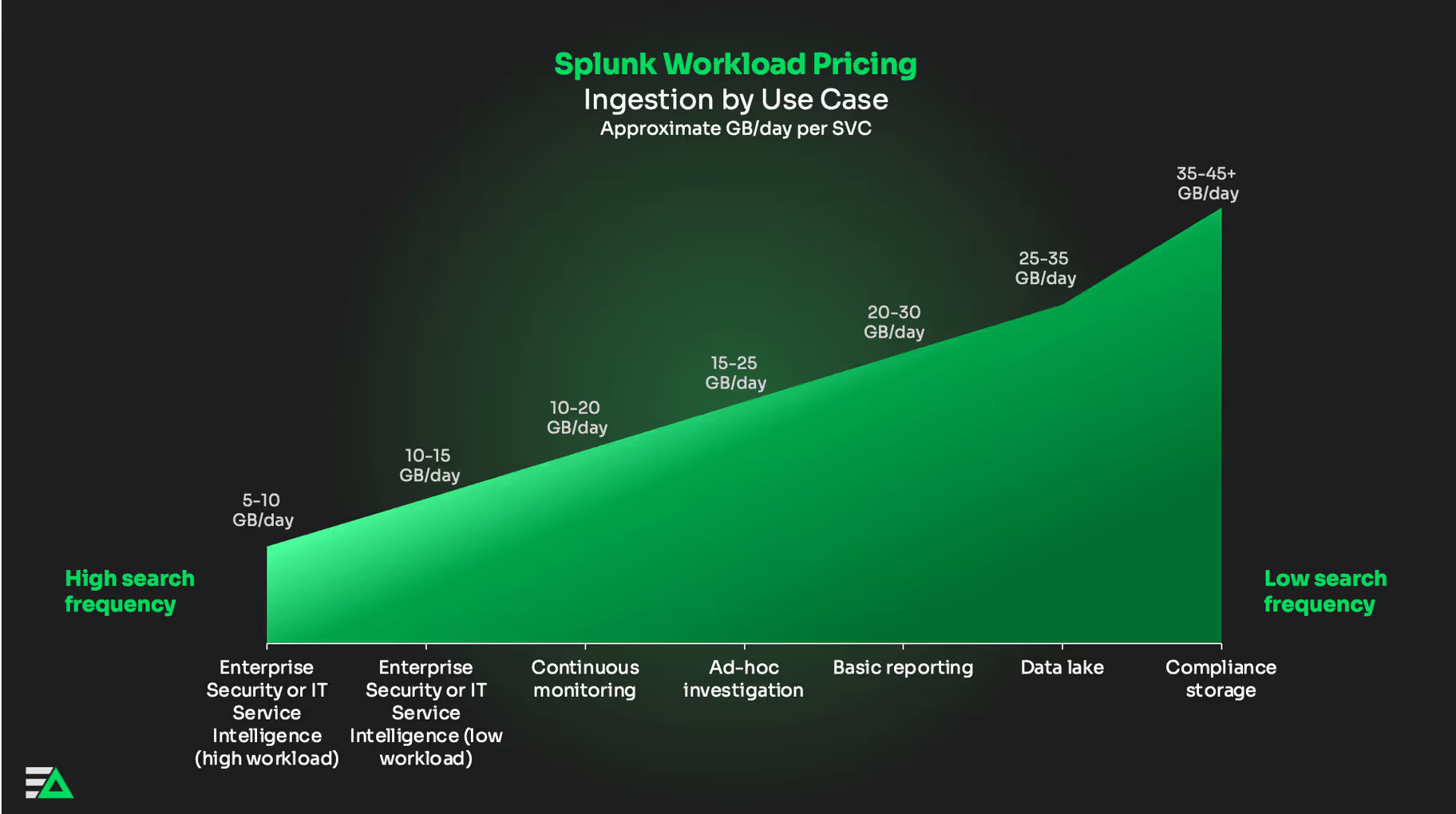
Enterprise Security, IT Service Intelligence (ITSI), and continuous monitoring use cases naturally consume Splunk SVC unit credits faster, given their compute-intensive nature. That’s because these use cases require customers to continuously run high-volume batch processing jobs on raw datasets.
Take, for example, a customer running a dashboard with nine panels. Every time a user renders or views the dashboard, each of the nine queries:
- Pulls all the associated raw datasets from indexes
- Filters out unneeded data
- Begins crunching raw data to create analytics
When there are a high number of dashboards, running those queries can be extremely taxing on the system, therefore driving compute and SVC consumption. This is especially true when dashboards are viewed or loaded concurrently. The same logic applies to more complex alerts running every few minutes. Here, you’re processing raw datasets when the underlying query runs, causing a spike in compute.
If your team consumes all available SVC credits, you’ll face overages. Additionally, you won’t be able to run another query until all other active queries are complete.
By charging for Storage Blocks, Splunk has decoupled your compute needs from your storage needs. In essence, you can purchase Storage Blocks to account for however long you’d like to retain your data.
How Will Moving to Splunk Workload Pricing Impact Your Bill?
When quantifying the impact of moving to Splunk Workloads Pricing, there is no one-size-fits-all solution. It ultimately depends on the data sources you are monitoring with Splunk Cloud.
That said, you should not expect a lower Splunk bill. The volume of data you ingest into Splunk Cloud strongly correlates with SVC utilization. That is because the ingestion process consumes a significant amount of compute resources. As a result, we’ve seen customers continue to neglect datasets and sample log lines after making the switch.
In many cases, customers migrate from Splunk Enterprise to Splunk Cloud. If you fall into this category, you should expect the initial ingestion process to create a spike in compute. Once your data is in Splunk Cloud, ongoing dashboards and real-time queries on large datasets can exhaust your SVC credits.
How You Can Use Edge Delta to Lower SVC Costs
Since the launch of Splunk Workload Pricing, we’ve seen customers use Edge Delta Observability Pipelines to streamline adoption. Here are a couple of examples you can apply to your Splunk Cloud deployment.
Lowering Ingestion-to-SVC Ratio
Edge Delta uses distributed stream processing to analyze data as it’s created at the source. In doing so, Edge Delta uncovers insights, statistics, and aggregates that are streamed to Splunk in real-time. When you need raw data – like when an anomaly occurs – Edge Delta dynamically routes full-fidelity logs to your preferred observability platform. This approach dramatically lowers the volume of data you need to ingest into Splunk, which in turn alleviates your SVC needs. How?
First, as we covered above, the ingestion process is one of the biggest drivers of compute consumption. Reducing ingestion volumes by nature lowers your compute needs.
Additionally, Edge Delta plays a massive role in optimizing dashboard load times and query performance, ultimately reducing SVC usage. It does so by pre-processing the raw data into datasets that are easily digestible and queryable in Splunk, and making query results natively available. As a result, customers can reduce a five-minute query on raw logs into a five-second query on optimized data.
Integrating New Services and Data Sources
By streaming your raw data through Edge Delta before ingesting into Splunk, you are ultimately optimizing the dataset – and clearing capacity to monitor more systems. As a result, Edge Delta has allowed customers to onboard new services and data sources into their Splunk deployment, gaining 100% observability without the need to purchase additional SVC units. In other words, you no longer need to neglect datasets.
Cutting Storage Block Costs
Multi-vendor data tiering is one of the most effective ways to reduce Splunk SVC licensing costs. Here, you can push different subsets of data to the ideal destination, based on how it’s used:
- Data that you use to populate dashboards can continue streaming to Splunk Cloud.
- Data that you need for compliance can move to a low-cost archive storage target, like Amazon S3, Microsoft Azure Blob, or Google Cloud Storage.
- Data that you query in an ad hoc manner can live in a cost-effective log search platform.
By storing raw data in these destinations, you can meet the unique needs of your team without requiring as many Storage Blocks as before.

By using Edge Delta in tandem with Splunk, you can expect to optimize your SVC consumption by 30-60%. With this level of optimization, customers have been able to ingest data from new sources without procuring more SVC credits. This combined value is why companies at all stages of SVC adoption are considering Edge Delta.